Characterizing Emergent Behavior in Synthetic Biological Circuits Using Data-Driven Biological Models
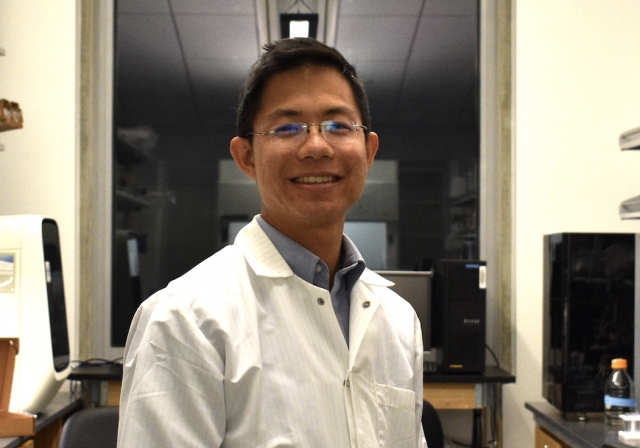
ONLINE CBE SEMINAR
All Spring 2020 CBE Seminars will be hosted online via Zoom. RSVP to receive zoom link by emailing info@bioengineering.ucsb.edu.
Zoom will open after the host has joined at the start of each seminar. You can ask questions through the chat forum and by raising your "hand" and the speaker will call on you.
Speaker
Enoch Yeung
Assistant Professor, Department of Mechanical Engineering
Center for Control, Dynamical System and Computation
BioMolecular Science and Engineering Program
University of California, Santa Barbara
Abstract
Biophysical systems often exhibit emergent behavior when engineered elements are incorporated. Hypothesis-driven models typically fail to reconcile these emergent dynamics when confronted with actual experimental data. An important and recurring example of emergent biological dynamics is the perturbed response of whole cell dynamics to the introduction of an endogenously engineered synthetic gene circuit. Existing biophysical models do not account for the circuit-to-host interaction, arguably the leading obstacle in building robust synthetic genetic circuits. Often these models are created independent of data to describe only genetic circuit components; they lack a mechanism for incorporating whole cell data. We improve on these hypothesis-driven models by constructing a learning process which takes advantage of the experimental processes involved in collecting RNA sequencing measurements. Specifically, we develop algorithms which approximate the Koopman operator, an infinite-dimensional operator that acts on observables to produce linear dynamics. RNA sequencing measurements are not handled well by the current state-of-the-art Dynamic Mode Decomposition (DMD) algorithms to approximate the Koopman operator. This is due to the data being high-dimensional, noisy, and typically containing a low number of timepoints. To overcome the difficulty of handling this type of data, we develop structured DMD and integrate it with the learning algorithm for sparse DMD, which is a recently developed form of regularized DMD that can handle sparse time-series data. Structured DMD mirrors the structure of the measurement collection process to identify the impact of adding synthetic circuit components to a host. We demonstrate that structured DMD can confront a large transcriptomics dataset (~0.1 TB) of a genetic NAND circuit, consisting of 29 engineered biological parts and over 7000 basepairs of synthetic genetic code. Most importantly, the algorithm reveals a first-ever glance into the whole-cell footprint of a synthetic logic NAND circuit in the organism Escherichia coli. .
BIO
Dr. Enoch Yeung has a B.S. in Mathematics from Brigham Young University, magnua cum laude with university honors and a Ph.D. in Control and Dynamical Systems from the California Institute of Technology. He has led many interdisciplinary research projects at the interface of synthetic biology and learning theory including the DARPA Synergistic Discovery & Design Program (currently serving as a performer and PI), DARPA Friend or Foe program (serving as co-PI), DARPA Living Foundries program, the 2018 High Performance Data Analytics Program, the NSF Molecular Programming Project, and the AFOSR Biological Research Initiative. He is an assistant professor in the Department of Mechanical Engineering at the University of California Santa Barbara. Previously, he served as senior research scientist in the Data Science and Analytics Group at Pacific Northwest National Laboratory and lead several internal research efforts in deep learning, network inference, and control of complex systems. His research interests center on learning algorithms for dynamical systems, control theory, synthetic and systems biology. He has served on several advisory panels for DARPA, NIST, the DoD SBME initiative, and the National Defense University. He is the recipient of Kanel Foundation Fellowship, the National Science Foundation Graduate Fellowship, a National Defense Science and Engineering Fellowship, the PNNL Project Team of the Year Award, and the PNNL Outstanding Performance Award.